Perception Subsystem
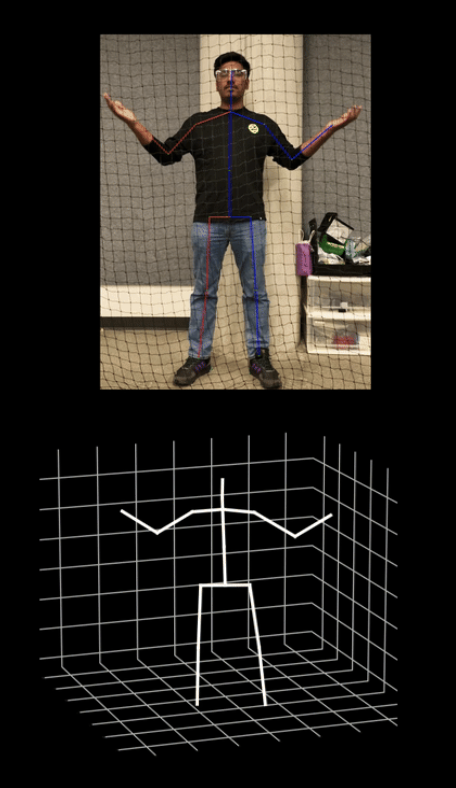
- Human Pose Detection Module
- The current Human Pose Detection Model we use is RTMPose, which takes the streaming images from the drone and outputs 2D pose (17×2 key points)
- Then, we use TransformerV2 to leverage these 17 2D key points into 3D key points.
- Human 3D Pose to LBS Motion Module
- An RNN network is currently trained using the dataset from Audio2Photoreal.
- The model feed takes the 3D Pose(17×3 Keypoints) and output LBS motion vector(104×1)
- Drone Depth Estimation Module
- We first use PoseFormer V2 and Zoe Depth to get the relative depth map and then estimate the distance between the human and the drone camera.
Human 3D Avatar Subsystem
- Decoder
- The decoder we used is provided by our sponsor, Meta.
- It renders the texture of meta codec avatars given lbs motion.
- The decoder itself is around 1-2 FPS.
- Renderer
- From the output of the Decoder, we could use the Renderer to generate images of the avatar given the camera pose.
- To achieve 30 FPS visualization, we downgrade the resolution of the generated avatar by 4x times, achieving 4 FPS for the decoder.
- Interpolation Module
- Due to the decoder’s inefficiency, we ran it every 8th frame and generated the frames in between using the interpolation method.
- With the interpolation, we could eventually achieve around 32 FPS.
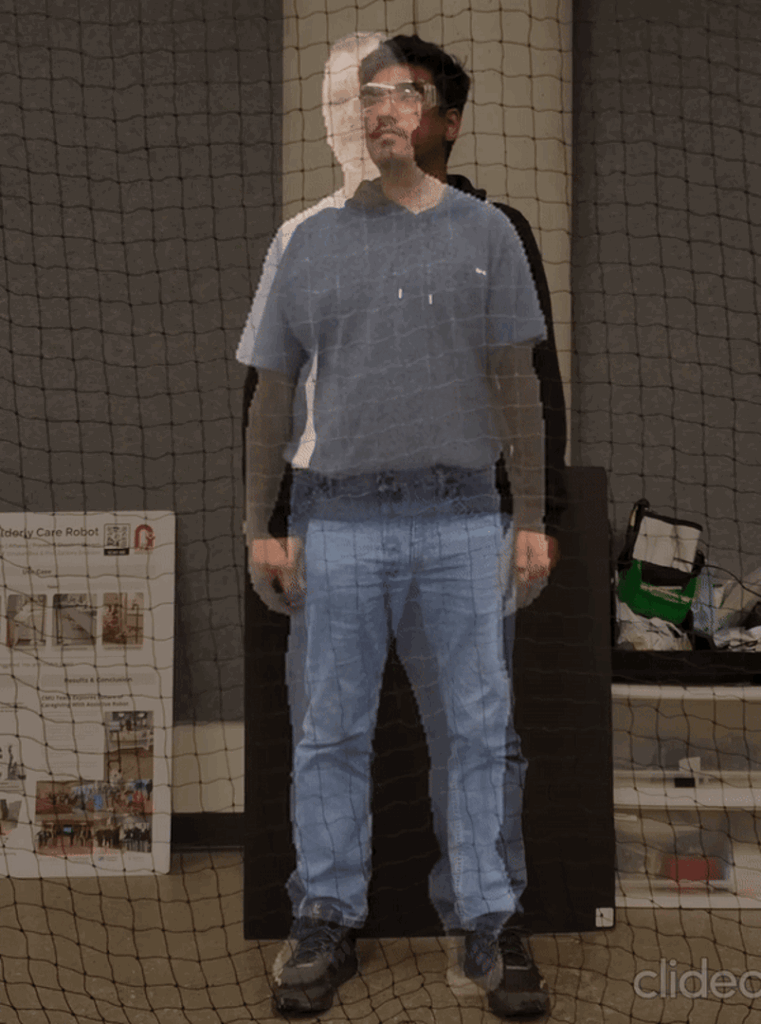
System Communication Subsystem
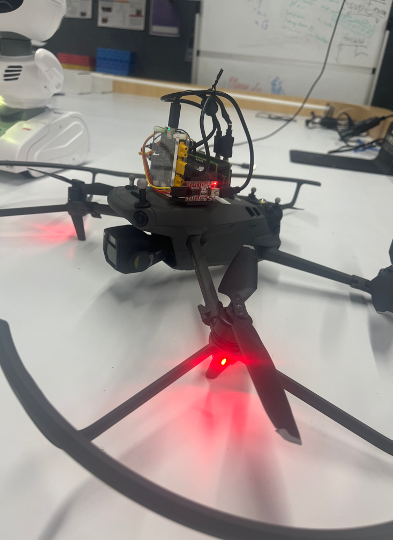
- ROS System for Perception Module
- We are using ROS nodes between each module to communicate and convey messages. Currently, we have a Video Capture node(Capture Drone Image through HD Capture Card from Remote Controller), a 2DPose Node(Detect 2D Pose and predict the LBS Notion), a Decoder Node(take the LBS motion and render avatar), and an Interpolation Node(take the rendered images and interpolate frames between them).
- Drone Control Module
- We can control the drone through Payload SDK using an e-port and an onboard computer.
- We have developed key points trajectory following and autonomously taking off, hovering, and landing.
Human Following Subsystem
- Yaw Maneuver with Head Pose:
- Leveraged head pose estimation derived from 2-D human pose detection to execute yaw maneuvers, enabling the drone to adjust its orientation to maintain visual tracking of the human subject.
- PID Controller for Pose Centering:
- Developed and implemented a Proportional-IntegralDerivative (PID) controller to maintain the human subject’s pose centered within the camera frame. While the PID controller operates smoothly within a speed range of 0.1 to 0.4 radians per second, it exhibits instability and jittering behavior beyond this range, necessitating further optimization for enhanced stability across variable speeds.
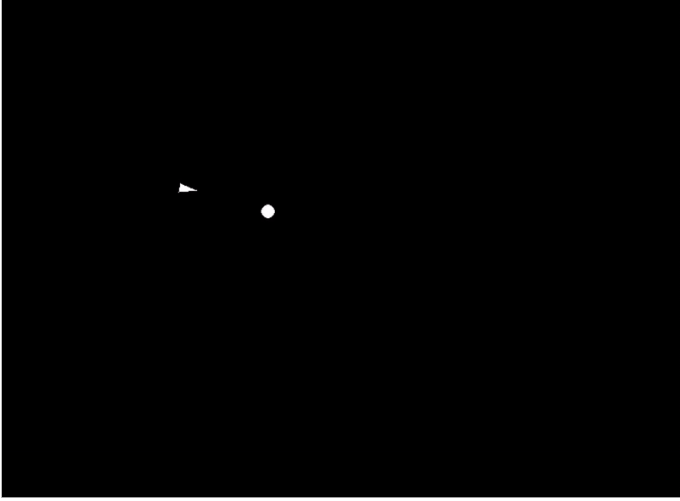
VR Headset Subsystem
Windows ROS Receiver
After encoding the human pose to the LBS Keypints, the set of 104 keypoints are streamed
over the ROS 2 network from the Ubuntu System to the Windows system. This is essential due
to the fact that the Quest Link drivers are only released by Meta for Windows systems. Another
issue that arises due to a Windows system is used is that two different environments that are
required to implement the pipeline. This is caused by ROS 2 and Pytorch being incompatible
on the same environment.
VUER Renderer
This subsystem receives the 104 keypoints from the ROS 2 environment and renders it
using VUER renderer. The VUER Renderer was chosen after initially attempting to render
directly using Meta’s DRTK renderer. However due to difficulty in estimating the right transforms for each eye and overall latency in the pipeline, the VUER Renderer was used instead.
After the generated 3D mesh is pushed on the VUER interface, we can then stream it to VR Headset using quest link.