SLAM subsystem consists of three different components: RTAB-MAP, ArUco Markers, and a custom map built of the test environment. During the Fall Semester, a detailed map of a section of the NSH 4th floor (see ”Generated Map – RTAB” as figure below) was meticulously generated through manual measurements of obstacle placement and dimensions, as well as the overall test area. This custom map served as the foundation reference for RTAB-MAP itself (see ”Custom Map” in Figure 11) and its mapping and localization capabilities.
Subsequently, over 30 ArUco markers were placed throughout the environment to act as visual landmarks. The markers were a key component to the success of the SLAM sub-system for the Unitree robot, as these markers helped to improve the localization accuracy. They played a key role in refining localization accuracy by offering distinct visual features that could be easily detected and tracked by the RealSense camera. The markers combined with visual odometry within the RTAB-MAP framework not only supported the robustness of the system but also helped to reduce any drift over time. This integrated approach using RTAB-MAP, ArUco markers, and a custom map proved to be a pivotal point in achieving sufficient mapping and localization results within the testing environment used for the FVD.
In the testing of the mapping portion of SLAM, this was done through visualization of the map generated and comparing it to the data expected to be seen. To test the localization and verify accuracy of output (adherence to the performance metrics) we collected ground truth data by manually measuring the environment to the centimeter level. This data was then used to compare against the localization results outputted by the SLAM algorithm. We conducted multiple test runs along the test setup, and performed data processing.
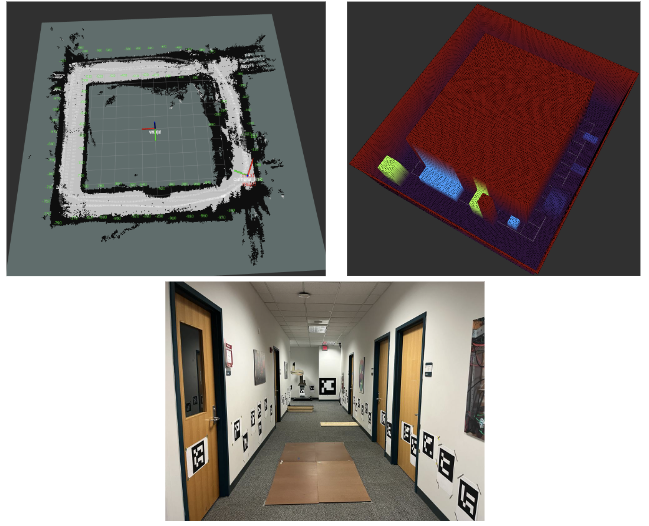
The map created from the RTAB-Map and the custom gridmap were aligned well as seen below.
Some Information regarding the individual algorithms are described below.
RTAB-Map
The figure above shows the progress made with the implementation of the base navigation stack on the Locobot (wheeled platform). This visualization was completed through handheld mapping which involved setting up the RTAB-Map on the lab computer and building it from the source. After installing the RTAB-Map, the SLAM algorithm was integrated with ROS (by base software developers) so interfacing with the Realsense viewer and visualization software did not cause any issues. After the implementation, the handheld mapping was tested by manipulating the realsense camera directly and a partial map of the environment (MRSD Lab) was visualized.
ArUco Markers
Over 30 ArUco markers were strategically placed throughout the environment to act as visual landmarks. Locations of the prime ArUco markers were manually measured and the nearby ArUco markers were calculated using the relative poses of the prime ArUco markers.
Kimera SLAM
During the initial few weeks, we implemented the RTAB-Map system; we later transitioned to the Kimera SLAM system. All the associated modules, including Kimera-VIO, KimeraRPGO, and Kimera-Semantics, were successfully integrated. The initial testing phase employed the uHumans dataset to evaluate the performance and compatibility of the integrated modules.
Subsequently, the RealSense D435i sensor was incorporated into the system and further tests were conducted in a variety of environments, such as the NSH basement, the A-level floor, and the MRSD Lab as shown in Figure 11. These diverse testing environments helped to ensure the robustness and adaptability of the Kimera SLAM system under different conditions.
In addition to the core Kimera modules, a MobileNet based semantic segmentation model was implemented and fused with Kimera-Semantics to enhance the system’s understanding of the environment. This process involved utilizing a pre-trained semantic segmentation model for the implementation of the module. To ensure optimal performance, the model was optimized using TensorRT, ultimately achieving a frame rate of 15 fps.
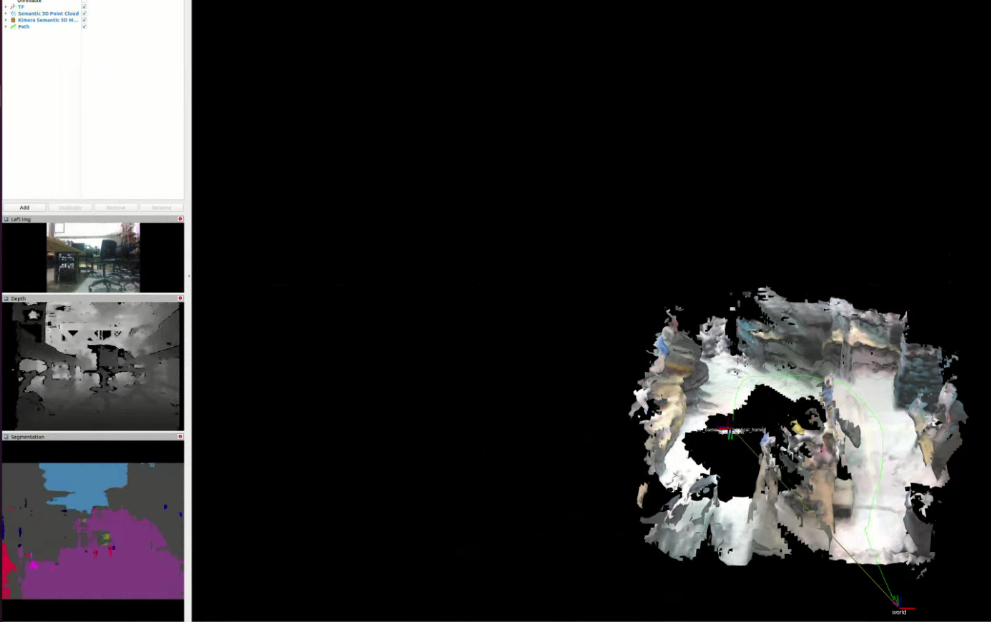
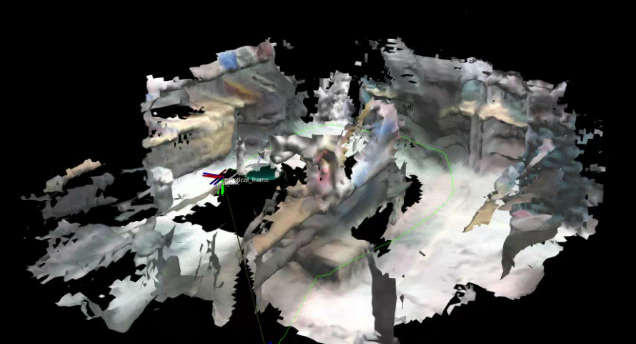
By integrating the Kimera SLAM system and implementing the semantic segmentation algorithm, the SLAM subsystem has been significantly enhanced, enabling it to more accurately map and localize the robot within its environment. During the Fall semester, further extensive testing, optimization, and integration will be carried out to ensure seamless compatibility with the planning stack. Additionally, a more suitable semantic segmentation model will be selected to better adapt to our specific environment.