Overview
The modeling subsystem provides a model for generating realistic behavior for agents at a road intersection inside the simulation. It tries to learn realistic behaviors from a combination of real-world and hand-crafted simulated data. The model replicates the traffic behavior from the trajectories extracted by the preprocessing subsystem. This model is also known as Optimal policy in the Imitation and Reinforcement Learning domain. It will also provide tunable parameters to observe certain behaviors more often, hence making the simulation platform more suitable for autonomous vehicle testing. The system is not “learning how to drive” rather it is trying to model a traffic scenario at an intersection for testing a self-driving vehicle. Hence we assume that the entire world state is known to the subsystem.
System Implementation Details
There are two parts of our behavior modeling subsystem, rule-based and learning-based modeling as shown below. Rule-based modeling learns high-level behaviors from real-world data such as traffic light breaking percentage etc. Learning-based modeling uses machine learning to imitate interactions and real-world trajectories.
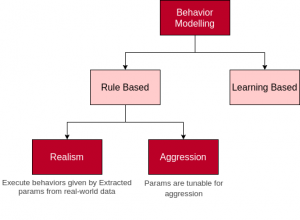
Modeling Branches
Rule-Based Modelling
Rule-based modeling provides two features, one is realism and the other is the aggressive tuning of the simulation. In rule-based modeling, we configure the parameters of our simulation to observe different behaviors. If the configured parameters are extracted from real-world data, the behaviors will be realistic and if the configured parameters are extreme values, it will make the simulated traffic behavior aggressive. As given in Data Processing Section, we are interested in configuring the following two high-level behaviors
- Traffic Light Violation Frequency [0%-100%]
- It shows on average how often do the vehicles violate traffic light in an entire simulation.
- The higher its value, the more aggressive the cars will be in jumping traffic lights.
- Minimum Leading Vehicle Distance [4m – 10m]
- This is the minimum distance that the rule-based model will try to maintain through-out the simulation. Sometimes it can get violated if the traffic behavior is aggressive such as when vehicles are jumping red lights.
- The higher this value the more spaced the cars will be during the simulation, lower values mean that the cars can get pretty close to each other.
- There is a lower limit to this parameter because the distance is measured from the center of the vehicle. Lesser than 4m means the vehicles will practically be in a collision.
In order to observe specific traffic behavior, we have made a configuration file that can be configured by the user in the following format
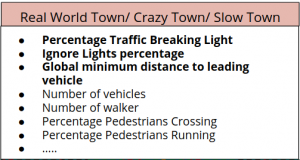
Custom Traffic Behvaiors
As we can see there are several other parameters that can be configured for more diverse behaviors but for evaluation purposes we have restricted ourselves to only two parameters as mentioned above.
In order to implement the rule-based model in Carla, we are using the Traffic Manager API of Carla. The traffic manager enables us to execute certain behaviors that can be easily configured by specific parameters. We extract those behaviors from the Data Processing subsystem and pass them to the Traffic Manager which executes those behaviors inside the Carla simulator as shown below.

Rule-based Modelling
In order to verify whether the executed behaviors match our requirement M.P.7, we extract corresponding parameters from the simulated ground truth trajectories. We are evaluating the following two behaviors.
- Traffic Light Violation Frequency
- Minimum Leading Vehicle Distance
Learning Based Modelling
This subsystem involves learning a model that replicates realistic behavior from the trajectories extracted by the Data Capture subsystem. This model is also known as Optimal policy in the Imitation and Reinforcement Learning domains.
Before going into the details of the modeling piece, we define a few terms-
- Ego Vehicle – A vehicle actor under consideration whose behavior is observed with respect to the environment in a particular episode of the training model.
- Actor- An entity in the traffic that is dynamic and influences the decision of the vehicle behavioral model. Currently, the following entities are treated as an actor in our system:-
- Vehicles
- Pedestrians
- Traffic Lights
- Environment – The environment constitutes the following aspects of the simulation:-
- All actor states except the ego vehicle.
- Lane Boundaries. This inherently takes into account the location of buildings and static obstacles in the town map.
- Road area
- World State- The ego vehicle and the environment state together constitutes the world state.
Conditional Imitation Learning Model
The current behavior modeling subsystem is based on the Conditional Imitation Learning framework provided by the Learning by Cheating (LBC) Paper. We use the privileged agent of LBC, which has access to all the privileged information, i.e. it can directly observe the environment (simulator state) through a spatial map. The spatial map M, is of a fixed dimension (W∗H). It contains the information 7 binary layers of positional information(M∈{0,1}W×H×7). The 7 layers includes lane boundary, road area, traffic light state, car positions, and pedestrian positions. The map is anchored at the ego vehicles’ current position,hence at each instance we get the state of all relevant objects in the ego vehicles vicinity.The task of the model is to predict K future waypoints [w=w1,w2,….,wk] that the vehicle should follow. The model also uses a high level “target” as an input parameter. The model is designed to predict a heat-map for the target points. We then obtain the top 4 “hottest” points in this heat-map to obtain the trajectory points using a spatial soft argmax.
The model is trained using behavior cloning from a set of real-world driven trajectories {τ0,τ1, …}. The data collation unit converts the data coming from Data Processing subsystem and stores each trajectory τ in the format τi={(M0,x0,R0,G0),(M1,x1,R1,G1)…}. Each trajectory contains the ground-truth map Mt, the agent’s position xt, orientation Rt in world co-ordinates, and the target/goal (Gt). We generate the ground-truth waypoints from future locations of the agent’s vehicle. Given a set of ground-truth trajectories and waypoints, our training objective is to imitate the training trajectories as well as possible, by minimizing the L1 distance between the future waypoints and the agent’s predictions. This is also shown in the picture below.
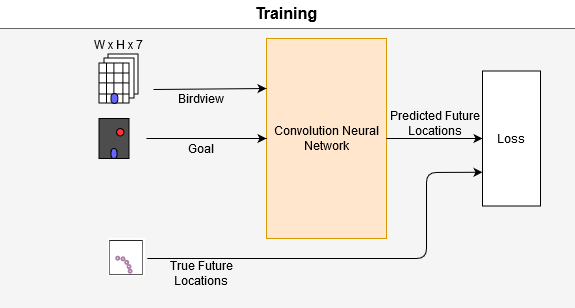
The actual CNN architecture varies from the LBC paper. We extend a newer version of the LBC model architecture provided by the author. It uses the DeepLabV3 Segmentation model with a Resnet backbone. We replaced DeepLabV3 with a DeepLabV3+ based segmentation model. This provides us a higher accuracy in general. Also, the original paper uses a ResNet as a backbone for the DeepLab model. A ResNet is usually quite heavy and is slow to run. We replaced that with a MobileNetV2 model that runs much faster than the original model. We can see the visualization of the spatial map with the predicted points(in blue) in the image below.
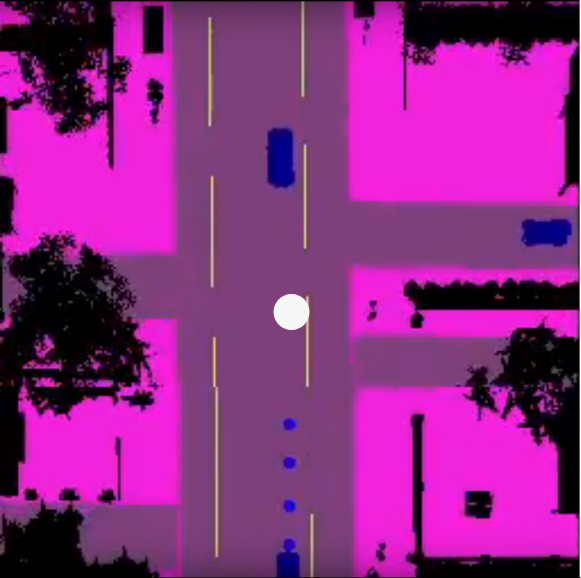
Analysis and Testing
Overall, our baseline behavioral model is capable of demonstrating general traffic behaviors such as,
- Responding to traffic lights
- Driving within lane boundary
- Following lead vehicle
- Collision Avoidance
- Switching lanes
Below we showcase a rare scenario, wherein the left we can see a car breaking red light in the real world and we have replicated a similar situation in the simulator. We can see that the LBC agent is capable of reacting to the vehicle in front by decelerating.
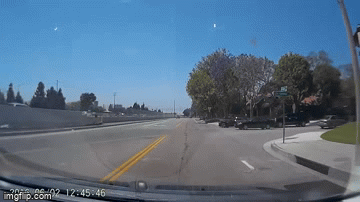
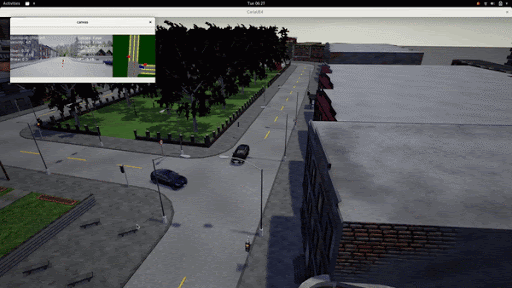
With our initial model in the Spring(the first half of the project), the model was quite slow to run and was also not optimized enough to run a large number of agents simultaneously. Our major goal with the learning based system was to optimize it both in terms of memory and computation speed. We updated the model architecture and trained the model on a dataset of scenarios provided in the 2020 Carla Challenge. Once this was trained, we evaluated the model individually against a held out train set and analyzed the qualitative results on the input dataset.
Strengths and Weaknesses
- Strengths
- The current rule based behavioral model is able to efficiently achieve the on-demand desired behavior of traffic light violation and minimum lead vehicle distance as configured by the parameters.
- Rule based behavior modelling also has the capability to observe other behaviors such as speed limit violation percentage, percentage of pedestrians jaywalking etc.
- The current learning based behavioral model is able to efficiently learn behaviors from the demonstrated data.
- It is also able to demonstrate general traffic behaviors such as following traffic rules, avoiding obstacles, and following leading vehicles.
- Weaknesses
- The learning based model can not generate specific scenarios on demand. Hence, there is a possibility of not observing certain behaviors at all in a scenario. We hope to overcome this by augmenting our behavioral model with probabilistic rule-based modeling and behavior trees.
- In learning based modelling the training was done using simulated data(generated in ). The expert trajectories were generated by a rule-based, fine-tuned, autopilot of the simulator. Hence the current model can only imitate a handful of behaviors produced by the autopilot. For different behaviors it is possible to retrain the Learning based model on varied behaviors(possibly real world datasets)
- The rule-based modelling simulation is prone to deadlock scenarios whereas if the traffic behavior is aggressive, too many cars jumping traffic light will create a deadlock at junction. In future, we can remove those cars which are static in a deadlock situation for a long time.